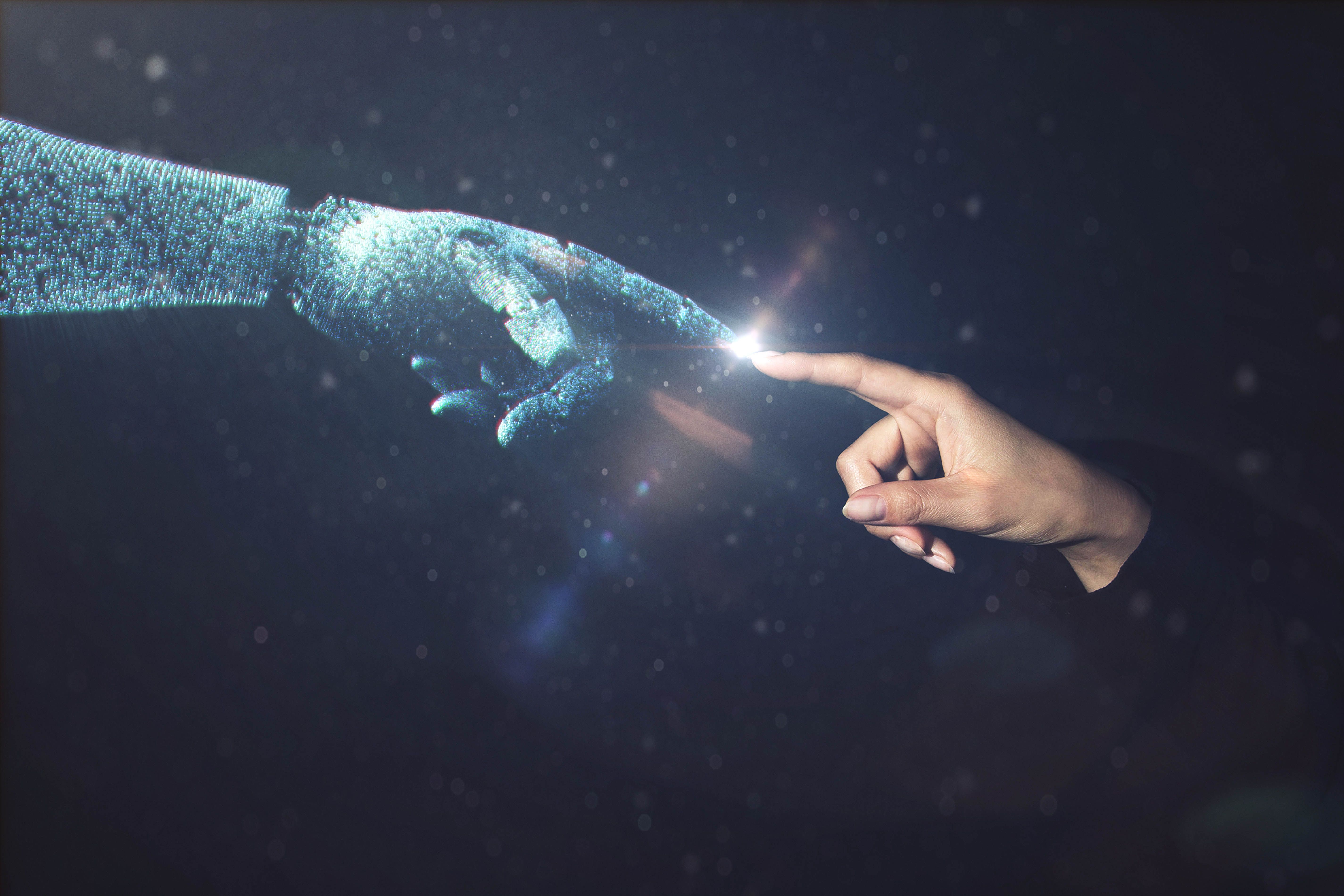
Embrace innovation by focusing on future trends rather than current circumstances. Many groundbreaking ideas have flourished following this approach – take Microsoft’s embrace of microprocessors, Salesforce’s utilization of cloud technology, or Uber’s dominance during the mobile era, for example.
As a researcher exploring the realm of Artificial Intelligence (AI), I’ve noticed an astounding rate at which Generative AI is advancing. This rapid progress poses a challenge: focusing on today’s capabilities could quickly become outdated. So, the question arises: can Web3, which has had a limited impact on AI’s evolution until now, adapt to the current industry trends and potentially shape its future?
2024 marked a significant turning point for generative AI as it saw groundbreaking research and technological breakthroughs. This year, the connection between Web3 and AI shifted from theoretical anticipation to hints of practical application. While early AI developments centered around huge models, lengthy training periods, massive computing systems, and deep corporate resources – making them mostly incompatible with Web3 – recent trends in 2024 are paving the way for meaningful collaboration between the two.
In terms of advancements in Web3-AI, the year 2024 was marked by numerous speculative projects, including meme-based agent platforms that mirrored optimistic market trends yet lacked practical real-world applications. As this hype subsides, a chance arises for us to reconsider the importance of practical applications. The landscape of generative AI in 2025 is expected to undergo significant transformations, with groundbreaking advancements in research and technology. These changes could spur the growth of Web3, but it’s crucial that the industry continues to innovate for future success.
Let’s examine five key trends shaping AI and the potential they present for Web3.
1. The reasoning race
The cutting edge for large language models (LLMs) is now centered on the development of reasoning abilities. Models such as GPT-01, DeepSeek R1, and Gemini Flash are emphasizing reasoning skills in their advancements. Essentially, reasoning empowers AI to simplify complex inference tasks into a series of organized, step-by-step procedures. This is frequently achieved through the use of Chain of Thought (CoT) methods. As with instruction-following, reasoning will soon be a fundamental skill for all leading language models.
The Web3-AI opportunity
When we talk about reasoning, it’s all about following complex processes that need to be both trackable and transparent. This is an area where Web3 really excels. Suppose you were reading an article written by an AI, and every step of its logical reasoning could be verified using blockchain technology. This would create a permanent record of the reasoning process. In a future dominated by AI-generated content in digital spaces, this level of traceability could become essential. Web3 can offer a decentralized, trustless system to verify the thought processes of an AI, filling a significant gap in today’s AI world.
2. Synthetic data training scales up
One essential factor fueling sophisticated thinking is the use of artificial data, often referred to as synthetic data. For instance, models such as DeepSeek R1 employ intermediary systems like R1-Zero to produce top-notch reasoning databases. These synthesized datasets are then utilized for refining the models, a process that lessens our reliance on real-life datasets and expedites model development while enhancing their resilience.
The Web3-AI opportunity
Generating synthetic data can be efficiently handled by multiple parties at once, making it perfect for distributed systems. With a Web3 architecture, nodes could be encouraged to provide their processing capabilities for synthetic data production, receiving compensation proportional to the utilization of their contributions. This could nurture a decentralized AI data market where both open-source and proprietary AI models benefit from synthetic datasets.
3. The shift to post-training workflows
Initially, AI models heavily depended on extensive preliminary training tasks that utilized numerous GPUs in thousands. However, systems like GPT-01 are moving towards intermediate and post-training phases, which allows for more specific functionalities such as advanced reasoning. This transition significantly changes the computational needs, making it less reliant on centralized supercomputers.
The Web3-AI opportunity
Instead of requiring large, centralized GPU farms during the pre-training phase, post-training can be distributed across a network that operates without a single controlling entity – a decentralized network. The emerging technology known as Web3 could enable such a decentralized environment for refining AI models, where contributors can invest their computational resources and receive rewards in the form of governance rights or financial incentives. This transition towards decentralization democratizes the process of AI development, making it feasible for more people to participate in creating and improving AI training infrastructures.
4. The rise of distilled small models
The technique of using big models to create smaller, task-specific versions, known as distillation, is becoming increasingly popular. Notable AI groups like Llama, Gemini, Gemma, and DeepSeek now offer efficient distilled variants, making it possible for these models to run smoothly on everyday hardware.
The Web3-AI opportunity
Compact distilled models can be efficiently run on standard consumer-level GPUs or CPUs, making them ideal for distributed inference systems. In a Web3 environment, AI inference marketplaces might arise, where nodes offer their computing power to process lightweight, compressed models. This would promote the decentralization of AI inference, lessening dependence on cloud services and enabling novel token-based incentives for contributors.
5. The demand for transparent AI evaluations
One significant hurdle in developing generative AI is the issue of assessment. Highly advanced models often seem to have learned existing industry standards so well that they become questionable indicators of actual real-world performance. When a model achieves exceptionally high scores on a specific benchmark, it’s frequently because this benchmark was part of the training data for the model. Currently, there are no reliable methods to validate evaluation results in models, causing companies to often rely on self-reported data in research papers.
The Web3-AI Opportunity
Utilizing blockchain technology for cryptographic validation could bring unprecedented transparency to the assessment of AI systems. By employing decentralized networks, it’s possible to independently verify model efficiency across common benchmarks, thereby minimizing trust in corporate assertions. Moreover, incentives within Web3 environments might stimulate the creation of novel, community-led evaluation metrics, elevating AI accountability to unprecedented levels.
Can Web3 adapt to the next wave of AI?
The evolution of Generative AI is experiencing a significant change in direction. Instead of just large, single models requiring extensive training periods, we’re now seeing new advancements like reasoning-focused designs, creative data collection methods, post-training enhancements, and model compression techniques that are dispersing the process of AI development.
In the initial surge of generative AI, Web3 took a backseat, but its growing influence presents exciting possibilities for decentralized systems to deliver genuine value. The pressing issue now is whether Web3 can adapt swiftly enough to capitalize on this opportunity and establish itself as a significant player in the AI revolution.
The opinions shared in this article belong to the writer and may not align with the perspectives of CoinDesk, Inc., its proprietors, or associated parties.
Read More
- INJ PREDICTION. INJ cryptocurrency
- SPELL PREDICTION. SPELL cryptocurrency
- How To Travel Between Maps In Kingdom Come: Deliverance 2
- LDO PREDICTION. LDO cryptocurrency
- The Hilarious Truth Behind FIFA’s ‘Fake’ Pack Luck: Zwe’s Epic Journey
- How to Craft Reforged Radzig Kobyla’s Sword in Kingdom Come: Deliverance 2
- How to find the Medicine Book and cure Thomas in Kingdom Come: Deliverance 2
- Destiny 2: Countdown to Episode Heresy’s End & Community Reactions
- Deep Rock Galactic: Painful Missions That Will Test Your Skills
- When will Sonic the Hedgehog 3 be on Paramount Plus?
2025-02-25 18:42